AI's meaning-making problem.
Meaning-making is one thing humans can do that AI systems cannot. (Yet.)
Hello friends,
tl;dr: Current discourse focuses on AI’s growing ability to generate human-like outputs. This overlooks human meaning-making: the ability to make subjective decisions that AI systems can’t make, but which are invisibly essential for making and using AI systems. Understanding meaning-making as something AI systems cannot do at all (for now) makes it easier to develop, productize, and deploy good AI systems.
This article builds on an earlier piece about meaning-making. I’m sure there’s lots of still-broken thinking here — so please send me your thoughts about what’s unclear and what I got wrong. (There’s a button for that at the very end.)
What can AI systems do?
Lots of AI discourse seems to be about what AI can do. This discourse shapes strategic decisions about how the AI ecosystem is developing: What foundational research to fund and engage in, what kinds of systems to build, where and how to deploy AI, how to write AI policy and regulation. In this discourse, the question is usually framed as “Can AI systems produce outputs that look like outputs from humans?”
For more and more types of outputs, the answer to this appears to be “yes.” AI systems can generate a sketch that a human could plausibly have drawn, or a contract that a human could have written, or a video that a human could have made, or recognise defective bananas the way a human could have.
Because AI systems seem capable of producing such a wide range of human-like outputs, it is nearly impossible to use this as a frame for making good decisions about how to shape the AI ecosystem. In other words, the question “Can AI systems produce outputs that look like human outputs?” isn’t discriminating enough to give clear direction when we have to choose what kinds of AI systems to build, how we should put them to use, what policies to adopt concerning them, or how we should regulate them.
We should focus instead on asking on a different, better question: “What can humans do that AI systems cannot?” Asking and answering this question makes it easier to make all those choices. I’ll be writing more about that in time to come. Today, I’m only focusing on answering the question, “What can humans do that AI systems cannot?”
What can humans do that AI systems cannot?
Humans can decide what things mean; we do this when we assign subjective relative and absolute value to things.
Sensemaking is the umbrella term for the action of interpreting things we perceive. I engage in sensemaking when I look at a pile of objects in a drawer and decide that they are spoons — and am therefore able to respond to a request from whoever is setting the table for “five more spoons.” When I apply subjective values to those spoons — when I reflect that “these are cheap-looking spoons, I like them less than the ones we misplaced in the last house move” — I am engaging in a specific type of sensemaking that I refer to as “meaning-making.”
Meaning-making is an everyday ability. It is so quotidian that most of us have stopped paying conscious attention to doing it except under particular circumstances (such as if we are judging a writing competition, making an investment allocation decision, or choosing just one out of 20 flavors of ice cream). There are actually at least four distinct types of meaning-making that we do all the time:1
Type 1: Deciding that something is subjectively good or bad. “Diamonds are beautiful,” or “blood diamonds are morally reprehensible.”
Type 2: Deciding that something is subjectively worth doing (or not). “Going to college is worth the tuition,” or “I want to hang out with Bob, but it’s too much trouble to go all the way to East London to meet him.”
Type 3: Deciding what the subjective value-orderings and degrees of commensuration of a set of things should be. “Howard Hodgkin is a better painter than Damien Hirst, but Hodgkin is not as good as Vermeer,” or “I’d rather have a bottle of Richard Leroy’s ‘Les Rouliers’ in a mediocre vintage than six bottles of Vieux Telegraphe in a great vintage.”
Type 4: Deciding to reject existing decisions about subjective quality/worth/value-ordering/value-commensuration. “I used to think the pizza at this restaurant was excellent, but after eating at Pizza Dada, I now think it is pretty mid,” or “Lots of eminent biologists believe that kin selection theory explains eusociality, but I think they are wrong and that group selection makes more sense.”
When I engage in meaning-making, I am making subjective decisions which other people might make differently. I would not go to East London tonight to meet Bob, but Bob will have other friends who would travel the same distance or further to meet him. For some collectors, there is no doubt that a Hirst painting is better than a Hodgkin (even if Hirst may be stretching the facts on when some of those paintings were made). And so on. Meaning-making is about subjective choice, which is why it underlies judgment, aesthetics, taste, and fashion. Everyone does it, but not everyone does it equally well and equally consciously.
The human ability to make meaning is inherently connected to our ability to be partisan or arbitrary, to not follow instructions precisely, to be slipshod — but also to do new things, to create stuff, to be unexpected, to not take things for granted, to reason. These qualities are unevenly distributed in the human population, but they don’t exist in AI systems at all except by human accident and/or poor design.
AI systems can’t do meaning-making … yet.
Last week, I asked ChatGPT to summarise a long piece of writing into 140 characters or fewer.
This is what happened: My first prompt was imprecise and had little detail (it was very short). ChatGPT responded to that prompt with a first version of the summary which emphasized trivial points and left out several important ones. I asked ChatGPT to re-summarise and gave it clearer instructions about which points to emphasize. The next version contained all the important points, but it read like a tweet from a unengaged and delinquent schoolchild. I asked ChatGPT to keep the content but use a written style more akin to John McPhee, one of the best writers in the world. On the third try, the summary was good enough to use though still imperfect (obviously it sounded nothing like John McPhee). I edited the summary to improve the flow, then posted it on social media.
For ChatGPT to produce this summary required a lot of hard-to-see meaning-making work:
Making the subjective decision about which points should be included in the summary and which should be excluded because they’re not important enough (Type 2 and 3).
Making the subjective decisions that a) the written style of the second version was wrong and inappropriate (Type 1), and b) that John McPhee’s written style would be more appropriate (Type 3).
Making the subjective decision that the third version would be useable with editing (Type 1).
Making the subjective decision that it would be faster, easier, and better to do the last edit than to try and reword the prompt to get a better outcome from ChatGPT (Type 2 and 3).
Making subjective decisions about word choice and order while editing the third version into the final posted version (Type 1).
All this meaning-making work was done by a human (me), because the AI system I was using couldn’t do any of it for me. This is generally true: AI systems in use now depend on meaning-making by a human somewhere in the loop to produce useful and useable output.
Meaning-making is quotidian and banal, so it’s easy to forget that we’re doing meaning-making while using AI systems that often seem magically advanced. So it takes constant reminders and active ttention to realise that much of the work AI systems currently seem to be doing when they produce human-like output is actually done by the humans working with the AI systems. It takes active effort to remember that AI is a tool that we have to know how to use — and the skill of using AI as a tool is largely built on the human user’s ability to make meaning.
And it isn’t only user meaning-making skills that crucial in the AI ecosystem. For now, highly skilled humans are still needed to make AI systems, and those skills are also largely built on meaning-making. In the trenches of the AI business, I bet it is even harder to recognize that the critical work being done is meaning-making work — but it is anyway. Some examples from obviously important areas are:
Fundamental research: When a research team sets out to construct a new AI model that outperforms existing models, they make a subjective decision about which approach to use in training it (Type 4), and subjective decisions about what data is worth including in or excluding from its training dataset (Type 1 and 2).
Productization: When a product team designs the user interface for an AI system, they make subjective decisions about user interaction modes and output presentation modes (Type 1 and 2), and about what controls to enable and prioritize (Type 2 and 3).
Evaluation: When an evaluation team writes a benchmarking framework or an evaluation framework for AI models, they make subjective decisions about what aspects of model performance they’re trying to measure, how they will operationalize those measurements, and what threshold of performance indicates “good” or “acceptable” (Type 3 and 4).
Policy and regulation: When a government policy team sets out to write guidelines for regulations governing the development of AI models, they make subjective decisions about what social and economic outcomes the regulations are meant to promote or avoid (Type 1 and 3).
In a nutshell, AI systems can’t make meaning yet — but they depend on meaning-making work, always done by humans, to come into being, be useable, be used, and be useful.
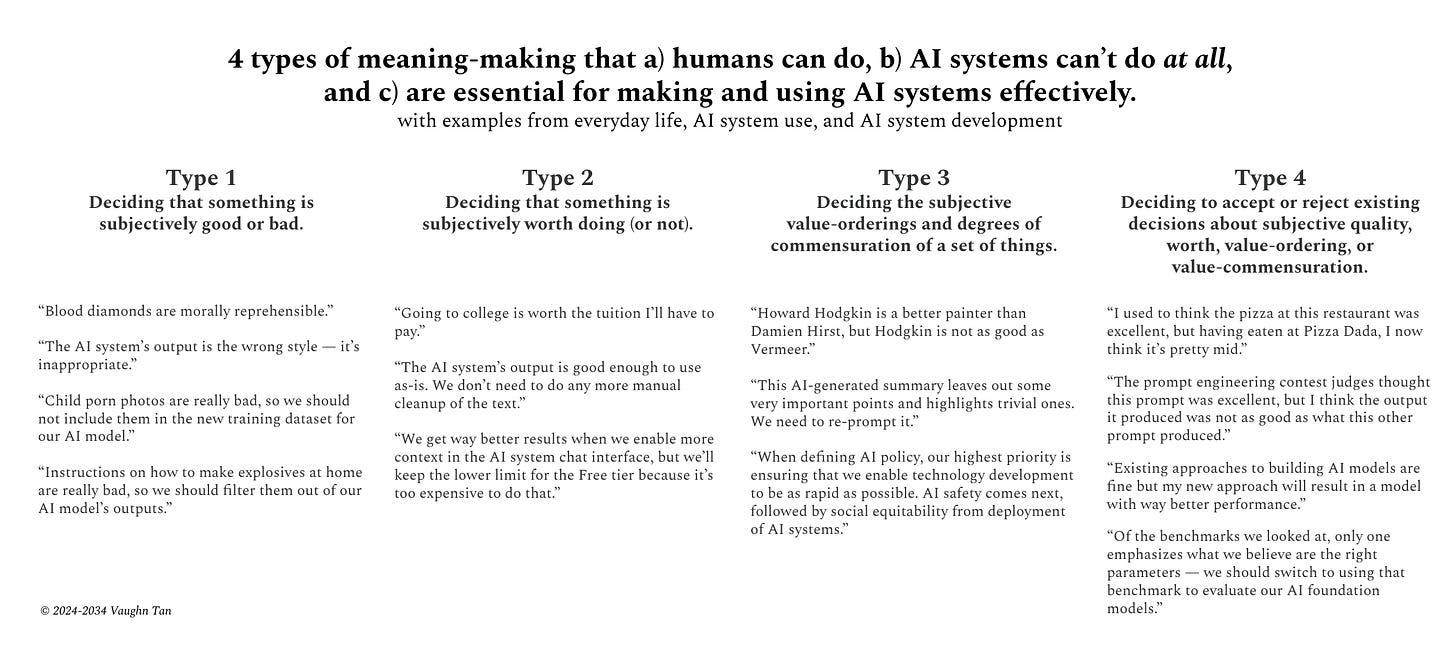
Next time: What AI systems can do better than humans.
See you here soon,
VT
The four types of meaning-making often occur together. For instance, it is often possible to think that something really bad (Type 1) is still worth doing (Type 2), as when the owners of privatised utilities systematically pay themselves massive dividends at the expense of investing in critical infrastructure).
can you redo the exercise but this time ask AI to make these judgment calls? i find that AI can definitely decide and definitely make judgment calls, but perhaps it is rather sophomoric and appears to lack taste. but perhaps another AI would disagree.
I came here via meaningmaking at work article
If we go beyond classification of good and bad, essentially meaningmaking is how you draw the boundary of a category or class, when we allow exceptions to the rules etc
In personality science, using factor analysis, there used to be 5 categories ie OCEAN
Now there might be 6 in HEXACO
A reptile is a cold blooded creature that lays eggs while a mammal is warm blooded that gives birth
But we put platypus a warm blooded creature that lays eggs under mammals
Then a more meta version of this would be how many classes / categories do we want? Is it a more qualitative or quantitative distinction?
Is one way of classification better than the other ? Under what circumstances?